Dobb·E
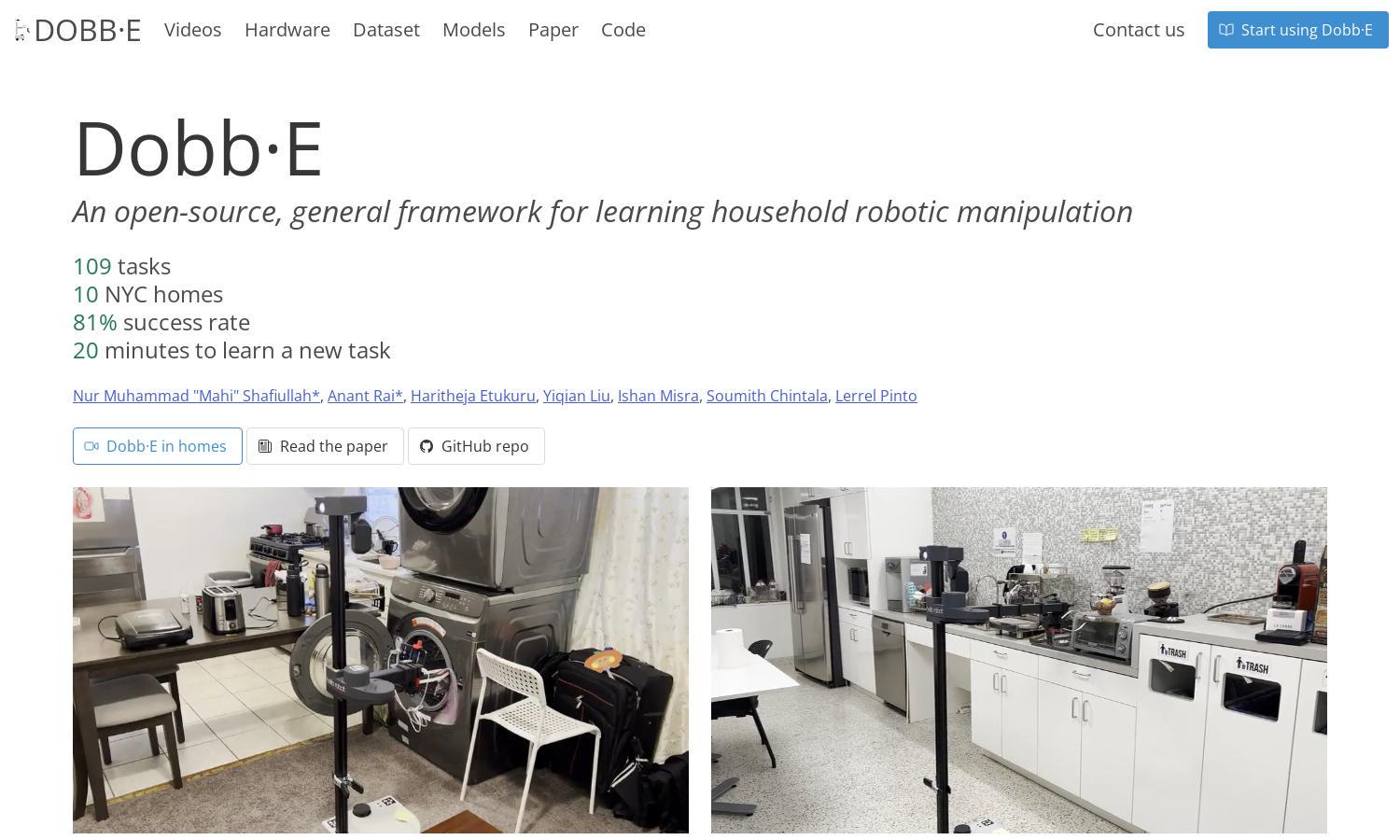
About Dobb·E
Dobb·E is a groundbreaking open-source platform designed to teach robots household tasks through imitation learning. By utilizing inexpensive tools and an extensive dataset, it allows robots to learn from user demonstrations within minutes, making it ideal for robotics enthusiasts and researchers aiming for practical automation solutions.
Dobb·E offers a free, open-source robotics framework without subscription fees. Users can access all features at no cost, fostering accessibility for developers, researchers, and hobbyists. This approach encourages innovation in home robotics, making advanced robotic learning accessible for everyone interested in smart home technology.
Dobb·E features a user-friendly interface that simplifies robot training processes. Intuitive navigation and clear documentation enhance the user experience, allowing users to easily collect demonstrations and train robots for home tasks. The seamless design supports both experts and newcomers in achieving successful robotic manipulations.
How Dobb·E works
Users begin their journey with Dobb·E by setting up the Stick demonstration tool, allowing them to collect data on household tasks. Then, they can utilize collected demonstrations to train the robot using the Home Pretrained Representations model. Within minutes, users can adapt and implement robotic solutions for various home tasks effectively.
Key Features for Dobb·E
Imitation Learning
Dobb·E’s imitation learning capability allows robots to acquire new home tasks rapidly. By leveraging user demonstrations captured with the Stick, Dobb·E instills adaptability, enabling efficient execution of various household chores, setting it apart in the home robotics landscape.
Homes of New York Dataset
The Homes of New York dataset is a unique feature of Dobb·E, consisting of 13 hours of real-world interaction data from diverse homes. This extensive dataset enables effective pre-training of robotic models, enhancing their ability to operate successfully in varied domestic environments.
Home Pretrained Representations (HPR)
Home Pretrained Representations (HPR) is a pivotal feature in Dobb·E, enabling efficient training for new tasks. By initializing the model with pre-trained capabilities, HPR accelerates the learning process, maximizing success rates in household task execution and optimizing robot performance in real environments.