MLflow
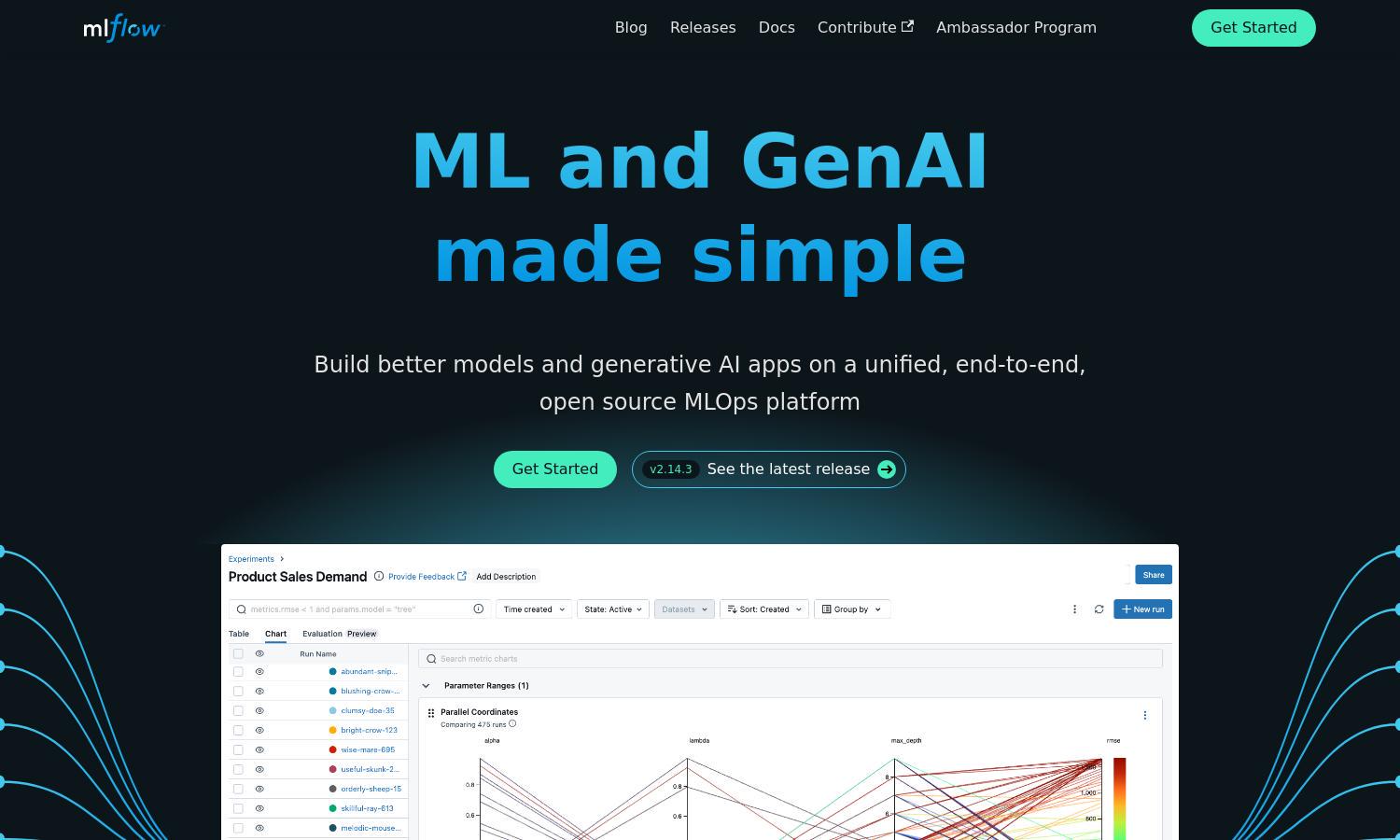
About MLflow
MLflow is a comprehensive open-source platform for managing machine learning and generative AI workflows. It streamlines the entire project lifecycle, from experimentation tracking to model deployment, making it ideal for data scientists and ML engineers. Its unique integration capabilities empower users to optimize performance effectively.
MLflow offers various pricing plans, including a free tier for the open-source platform and premium options providing advanced features and support. Upgrading to a premium plan allows users access to additional integrations, enhanced support, and greater flexibility in managing ML projects, improving overall productivity.
MLflow's user interface is designed for simplicity and efficiency, featuring a clear layout that enhances navigation through its various tools. Users can easily access experimentation tracking, model management, and deployment features. This user-friendly design significantly improves the browsing experience, making ML workflow management intuitive.
How MLflow works
Users interact with MLflow by first signing up and onboarding through a streamlined process, allowing them to set up their machine learning projects quickly. Once onboarded, they can navigate its powerful features, including experiment tracking, model registry, and deployment options, with intuitive controls, making it easy for users to manage and optimize their workflows seamlessly.
Key Features for MLflow
Experiment Tracking
MLflow's experiment tracking feature allows users to log, compare, and visualize various machine learning experiments efficiently. This function empowers data scientists to analyze results effectively, enhancing decision-making and improving project outcomes. With MLflow, experimentation becomes a streamlined process, helping teams achieve better results faster.
Model Registry
The model registry in MLflow provides a centralized repository for managing machine learning models, enabling version control, tracking model lineage, and facilitating collaboration among team members. This feature ensures that users can efficiently deploy and manage their models, significantly enhancing workflow and project collaboration.
Model Deployment
MLflow’s model deployment feature enables users to easily package and deploy their models at scale. With robust support for various deployment environments, MLflow simplifies the transition of models from development to production, ensuring users can deliver high-quality solutions quickly and efficiently, ultimately boosting their productivity.